Doomed to fail without AI?
EMO Hannover 2019: Applying an “engineering approach” to the systematic use of artificial intelligence
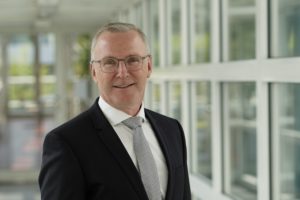
The headline is intentionally provocative. According to a 2018 study by Boston Consulting, China, Japan and the USA have been upgrading their artificial intelligence (AI) systems for some time now, meaning that Europe urgently needs to take action. Prof. Jörg Krüger, Head of the Industrial Automation Technology Department, at the Institut für Werkzeugmaschinen und Fabrikbetrieb (IWF) at the Technical University of Berlin, and Head of the Automation Technology Division at Fraunhofer IPK, is now making the necessary wake-up call. The AI pioneer’s tip for production engineers: come to EMO Hannover 2019 and be inspired by the WGP (Wissenschaftliche Gesellschaft für Produktionstechnik — German Academic Society for Production Engineering) and trailblazing machine manufacturers to take your first steps towards AI.
Professor Krüger, how and when did you did first come into contact with AI?
Jörg Krüger: That was in 1992 when I was exploring the use of artificial neural networks in the diagnosis of machine tool axes. Today I am fascinated by the tremendous advances made in deep learning (part of machine learning based on neural networks and large amounts of data, author’s note) and convolutional neural networks (according to Wikipedia, a machine learning concept inspired by biological processes, author’s note), some of which already exceed human capabilities in pattern and image recognition in the processing of audio and video data.
Mastering complex AI systems with simpler structures
In November 2018 the book Der unterlegene Mensch: Wir uns uns digitisieren mit Algorithmen, künstlicher Intelligenz und Robotern selbst weg? was published by Riva-Verlag in Munich. What do you think of the pessimistic views on AI expounded by the author Prof. Armin Grunwald, who, as head of the Office of Technology Assessment at the German Bundestag (TAB), has a certain amount of influence?
Jörg Krüger: Some aspects are becoming ever more complex and so I understand his scepticism regarding the inscrutability of these systems. We need to create simpler automation structures that facilitate better understanding of and control over the complexity of machine learning. The issue is whether production engineers can trust a self-learning system enough for them to deploy it in production. Education and training often don’t adapt quickly enough to such rapid developments in research. This means that only with a considerable delay are we able to acquire the skills necessary to master this complexity and build confidence in these new technologies. All this, of course, fuels the notion that humans will eventually be pushed aside.
Exploiting employees’ knowledge of their domain
And then the production engineers find themselves confronted with a mountain of Big Data: What should they do with this?
Jörg Krüger: It depends very much on what kind of data you’re talking about and what the production engineer’s objective is. One user, for example, wanted to use image recognition to identify and manage 50,000 to 60,000 parts in a warehouse. Previously, a warehouse worker would do the work of identifying and sorting the parts using a catalogue. We only had a limited number of images taken of each part, not enough to map a neural network structure. However, we found that by using pre-trained networks based on non-industrial image data in combination with a limited amount of industrial component data, it was possible to achieve acceptable recognition rates for providing assistance functions even from an early stage. The AI system then works as a semi-automatic assistant that displays the five most likely parts to the warehouse worker. Thanks to this assistance, he now works much more efficiently and accurately. But this only works if you know the processes very well. That’s why my message is: don’t just invest in hardware and software, you also need to make systematic use of the “domain knowledge” of the production staff. People must also learn to evaluate processes in the factory and decide which tasks AI can take over. The inclusion of domain knowledge from production is crucial in order to identify new areas of value creation potential quickly and systematically.
Data is digital gold dust
Smart assistance is one aspect, but what else does AI have to offer?
Jörg Krüger: The data generated by companies is digital gold dust for me. In my experience, many companies are not yet aware of just what value creation potential it holds. Machine learning tools are becoming increasingly powerful. In production, we should now be systematically combining data with domain knowledge in order to refine processes and make them more efficient. I’m interested in tackling this issue with colleagues from WGP. For example, we should no longer be focusing on increasing recognition rates with the help of machine learning methods as we did in the past. Instead we should be analysing the potential of existing production data for machine learning more systematically than before and exploiting the resulting potential for raising production efficiency levels more systematically. I recommend watching the YouTube video by the Canadian scientist Ajay Agrawal or reading his book Prediction Machines: The Simple Economics of Artificial Intelligence. Here, automation or production engineers view the opportunities of AI from an economic perspective in order to discover the digital gold dust in their own company. Completely new value creation models and niches suddenly emerge for small businesses, especially for start-ups.
What role will sensors play?
Jörg Krüger: “Sensorisation” is usually the first step in acquiring data for learning. The more powerful and cost-effective the tools for machine learning are, the more valuable the underlying data becomes. Especially in the field of sensorisation, great progress is being made in research and development in relation to Industry 4.0 — a good prerequisite for taking the next step towards machine learning in production.
Systematic AI solutions based on German “Ingenieur-Denke” (engineering thinking)
But what are our chances against countries like China, which are investing vast amounts in AI?
Jörg Krüger: The levels of investment in AI infrastructure, as we’ve seen in China in particular, are indeed impressive: it’s difficult to see how we could match that here in Germany. In terms of international competition, however, I see a bright future for Germany in applying structured engineering thinking to the industrial use of AI or machine learning. We should then be able to maintain and build upon our very good global position in the field of automation in the future.
What are you most interested in seeing at EMO Hannover 2019 – not only in your capacity as a production engineering researcher interested in AI?
Jörg Krüger: I’ll certainly be interested in seeing the exhibits being presented by our WGP colleagues. For example, I heard that an institute will be showcasing something very exciting in the field of pattern recognition on machine tool drives. That’s all I can tell you. It should also be worthwhile checking out the stands of some machine manufacturers and automation companies.
Professor Krüger, thank you for talking to us.
Trumpf: Using AI in production
“Artificial intelligence doesn’t kill jobs; it’s simply a further logical step towards securing Germany’s competitiveness and prosperity,” says Dr. Thomas Schneider, Head of Development at the Machine Tool Division of Trumpf GmbH & Co. KG, Ditzingen. “The mechanical engineering knowledge we have gathered over decades is essential for making use of artificial intelligence in industry. We must seize this opportunity.” In Swabia they are already deploying AI: 25 Trumpf employees are working to ensure the transparent and group-wide coordination of the company’s AI activities. Artificial Intelligence can, for example, use an automatic laser system to analyse the removal of cut sheet metal parts – initially unsuccessfully, but ultimately successfully – and automate the procedure using the resulting data. Trumpf can then apply this method to all machines of this type. AI has also proved successful in the company’s own production: sensors in the machine record large amounts of data during a short test and send them via the controller to the cloud, where the AI solution analyses them automatically. An ingenious combination of simulation, measurement and analysis methods enables the machine to be monitored in countless operating states. If there is a problem in the data, it not only recognises the error, but also knows how to correct it from its previous analyses.
Author: Nikolaus Fecht, specialist journalist from Gelsenkirchen
((Size: around 8,800 characters including blanks))